White Paper & Webinar: Combining machine learning with physics and chemistry models to accelerate materials R&D
The utilization of machine learning and artificial intelligence is currently playing a pivotal role in accelerating research in the fields of chemistry and materials.
It is straightforward to generate quantum chemistry inspired descriptors for a large set of molecules using the Amsterdam Modeling Suite. Our Python library, PLAMS, facilitates the conversion of SMILES strings into chemical descriptors, enabling the calculation of electronic properties with ADF or DFTB. In a collaborative project with Intellegens for the Henry Royce Institute, we have discovered that leveraging these chemistry-inspired descriptors can significantly enhance the design of superior dyes, as discussed in a recent webinar and white paper.
We are enthusiastic about tackling new challenges in prediction and design by harnessing the power of chemistry-inspired descriptors. Feel free to get in touch with us to discuss your research and development needs!
SCM has also recently developed molsg, a new module containing algorithms for parsing molecular structures as their substituent subgraphs. These subgraphs can be used for efficient molecular database searching, as descriptors in property modeling, for identifying identical (or similar) molecules from different 3D geometries, and for many more applications. Molsg has already been used to develop a new estimation method for sigma profiles in COSMO-RS, surpassing the performance of traditional QSPR models by nearly twofold.
We would love to hear if you have any problems where you think molsg could help you more efficiently search the chemical design space.
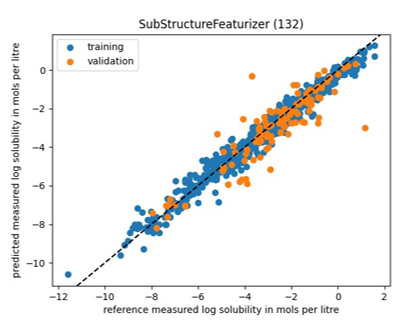
And don’t forget to subscribe below to our future webinar series!